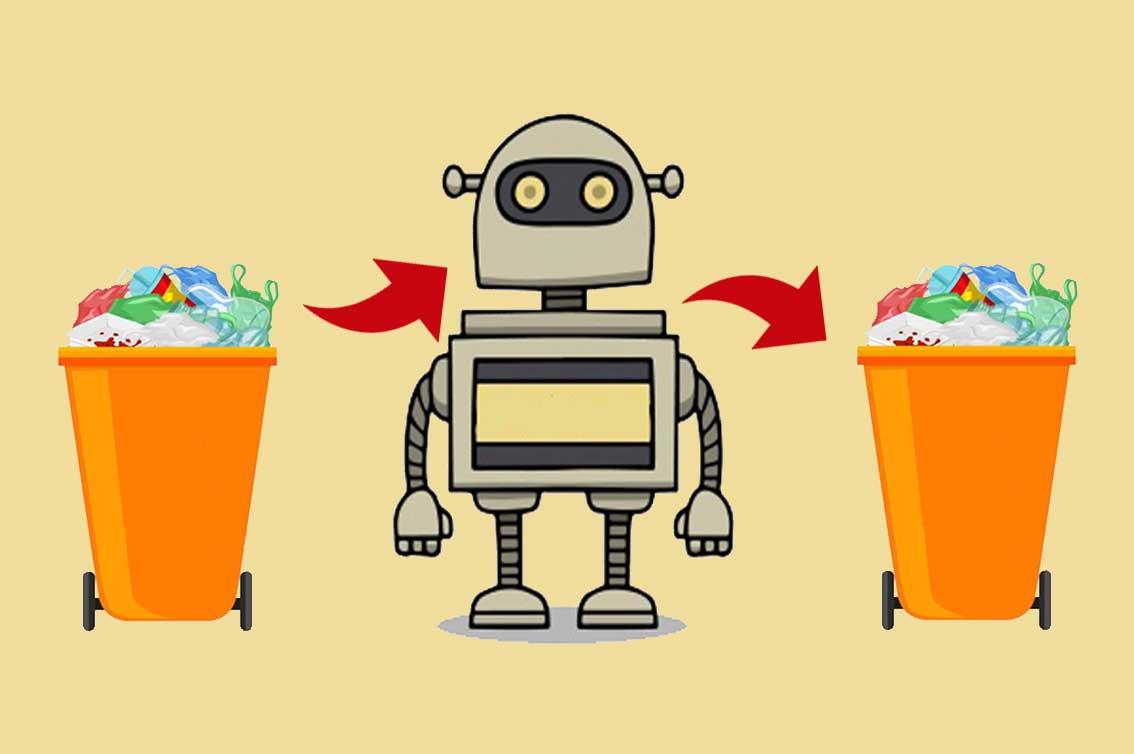
How to leverage neural machine translation in test item translation
by Pisana Ferrari – cApStAn Ambassador to the Global Village
Advances in artificial intelligence (AI) have opened up enormous opportunities in the field of translation. Today, with a well-designed – hybrid – workflow that combines human expertise and neural machine translation (NMT), it is technically possible to produce multiple language versions of test item banks faster and at a more affordable price than three years ago. If that is what you want to strive for, certain stages cannot be skipped.
Garbage in, garbage out
First of all, one has to remember that the output of MT engines is only as good as what is fed into it. For some language combinations and some domains, the result produced by some engines is sometimes impressive. But when it comes to test content, disambiguation is key. Without external help, the machine cannot infer from the context whether a “Dutch teacher” actually teaches Dutch or is merely a Dutch citizen who teaches any subject. Test developers will need to disambiguate source material and work together with linguists to prepare contextual elements for both human translators and MT engines to interpret.
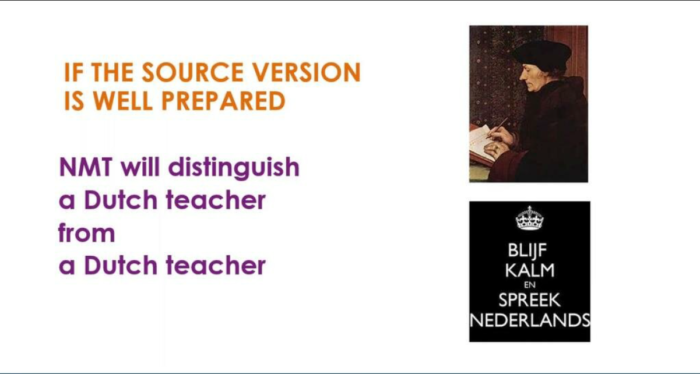
The mindset should be “upstream” work
What eats up a lot of time and resources is adding multiple reviews to reviews. Reviews by linguists, subject matter experts, proofreaders, stakeholders, censors, end users, bilingual staff at the client, you name it. If decent preparation work has been done before the translation begins, there can be more automation and less reviews during, and after, translation. More preventative linguistic quality assurance early in the process, less corrective action late in the process. Automated quality assurance checks increase consistency and take over repetitive tasks such as harmonizing quotation marks, checking whether all segments are translated, or checking adherence to a glossary. Item writers need assistance to “engineer” the source version to make it suitable for translation into other languages and to make it suitable for a man-machine translation (MMT) workflow.
How cApStAn can help you
This assistance is exactly what cApStAn has to offer to the testing industry. You need to make sure AI is fed with high quality input to harvest quality output. AI can leverage databases of known issues (and known workarounds). High quality input refers to both the source version of the text and to existing, validated translations that are fed into the system (“translation memories”). Interacting with linguists and cultural brokers to train your input data boosts efficiency. To train input data means to trim it, to program rules, to create style guides, to compile glossaries, to prepare routing, filters, dynamic text, and contextual information that can be read and interpreted by man and machine. This allows to diagnose issues and propose fixes before the MMT workflow kicks in.
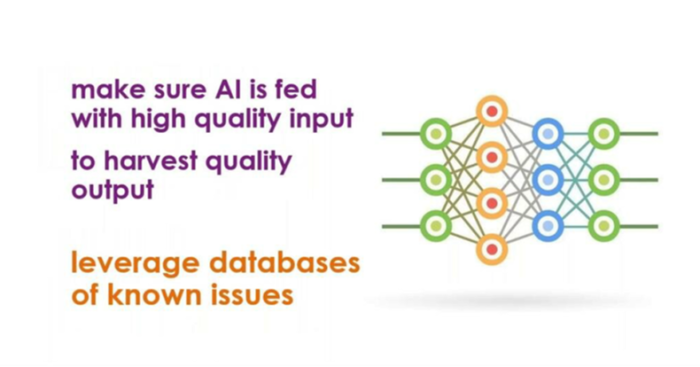
Augmenting the source version before translation
A sound approach should be proactive, collaborative and interactive. Spend some time and money reviewing and augmenting the source version together with linguists/culture brokers. The interaction between psychometricians, cognitive psychologists and linguists:
- Instils new dynamics in item writing.
- Produces a corpus of item-by-item translation and adaptation notes.
- Upgrade your item pools to translatable master versions, ready to input into AI.
- Train a good MT engine with high-quality translations.
- Enlist the help of experts to usefully supplement NMT in tomorrow’s Man-Machine Translation workflows.
- AI is an umbrella term for (developing) a computer’s ability to perform and resolve tasks that are generally thought to be rather easy for a human brain but really hard for a computer.
- “Machine Learning” (ML) is a subset of AI. ML is predictive: one feeds input data into algorithms that are programmed to use statistics and predict an output value (within an acceptable range). In translation, ML is used to identify recurring patterns in the human translator’s choices, which will help the system predict a higher likelihood for one possible translation versus another.
- “Deep learning” (DL), finally, is a subset of ML: these are ML algorithms with hidden layers. The programmers cannot predict how exactly the computer will use these. In an image recognition programme, for example, one just labels a 1000 pictures of oranges as “orange” and a 1000 pictures of apples as “apple” and the DL system will create its own rules to distinguish which is which, while in standard Machine Learning, the programmers write the rules that the computer uses to identify/recognise what is on the picture.
- NMT draws on DL to make decisions: it uses input such as lots of bilingual data to create its own fluency rules.
cApStAn’s “Translatability Assessment” is part our Linguistic Quality Assurance process. It helps identify any potential issues upfront, before translation begins, by checking test items against a list of translatability categories, and reporting potential issues in a centralised monitoring tool. Alternative text may be proposed without loss of meaning.
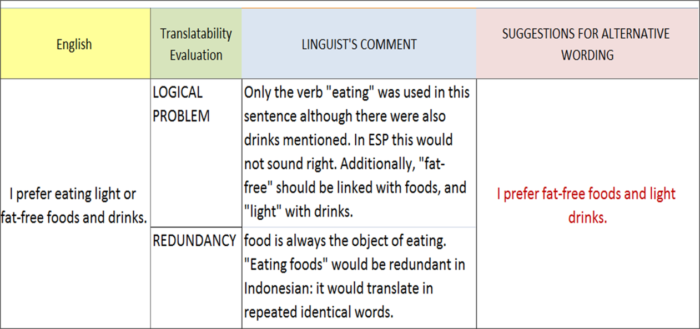
Read more about our Linguistic Quality Assurance (LQA) processes here
Action points for the testing industry
It is important to determine the skills that item developers (and human linguists) need to acquire/hone so that their expertise can effectively be woven into new MMT workflows.
If they can work with experts to define the new skills that linguists will need (translation technology, natural language processing, discernment) and test linguists for these skills, they will have a new product that will be in high demand in the buoyant localization market.
It is high time to:
If you do your homework before translation process begins, you can catch the early train of tomorrow’s multilingual assessment.
Understanding the key concepts
What is the ideal workflow to combine human expertise and Neural Machine Translation to produce multiple language versions of your test item banks?
What preparation work is necessary before translations begin?
What are the main action points for the testing industry?
Photo credit: Graphillus/Milan