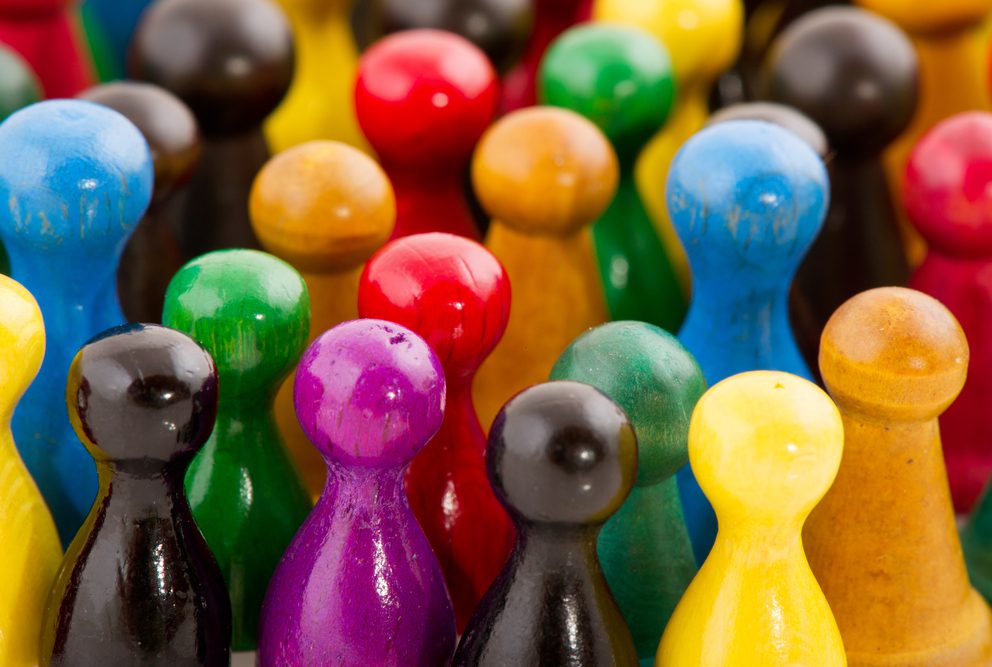
Including diversity in AI can help to minimise bias and also makes good business sense
by Pisana Ferrari – cApStAn Ambassador to the Global Village
Bias and discrimination are “creeping” more and more into day-to-day (automated) decisions on access to jobs, credit, parole and many more areas. In a recent talk at EmTech Digital, Tess Posner, Executive Director at AI4ALL, says that AI systems are only as fair and ethical as the data they’re trained and AI is currently being led by a limited and homogenous group of people in terms of gender and race. Only 13% of CEOs at AI companies are women and the numbers for minority representation are “abysmal”, not only in research but also in the broader engineering field. AI4ALL’s mission is to include diversity and inclusion in AI development: this not only serves to mitigate bias but also to maximise AI’s potential transformational benefit. They partner with top research institutions (Stanford, Berkeley, Princeton…) and companies to run education and mentorship programs in the U.S. and Canada that give high school students, including underrepresented ones, early exposure to AI.
Diversity can enrich the AI field by tapping on talents that could otherwise be missed, opening up to new and diverse sets of problems and leveraging the network effect of bringing in people from all walks of life. On the latter point, Posner reports that 90% of students having attended the AI4All programs get into the AI field and, for every student that goes through the program, they educate 11 more. Posner quotes a recent Intel report which puts at $500 BN the projected new value for tech industries that will result from improving diversity. So what can companies do, in practical terms, to improve diversity? Posner lists a few simple steps to take: 1) adopt existing standards, eg. the IEEE Global Initiative 2) include prevention of bias in the company’s code of conduct 3) introduce bias mitigation programs for engineering and product leads. Very importantly, AI products should be rigorously tested for bias before releasing and should be monitored for bias throughout their life cycle. And companies should go to the real “root cause”, ie. prioritising diversity in data inputs, in teams, in leadership. They should hire or train diverse AI talent from the start and track diversity metrics not just for hiring but also with the retention data.
The risk of not addressing the AI bias issue is that this could lead to mounting negative public opinion and this in turn could bring on “hastily crafted” regulation and halt some of the potential great innovations that we are all looking forward to. “The AI train has left the station and it’s moving fast. While this is true, it’s still early.” The time has come to act.
Link to talk: https://www.technologyreview.com/video/610704/addressing-bias-in-ai/
More about AI4ALl at: http://ai-4-all.org/