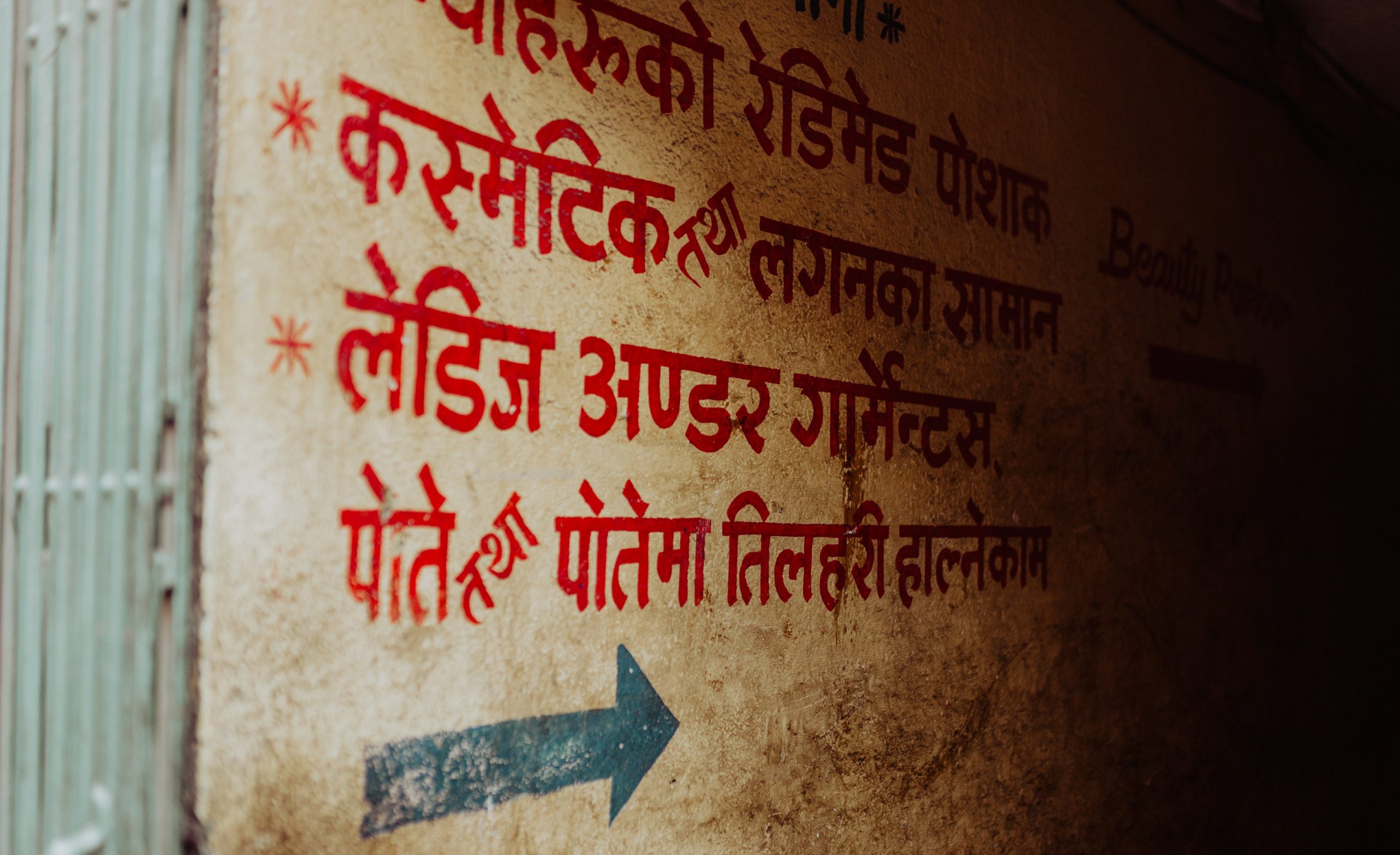
Promising research on machine translation for low-resource languages
by Pisana Ferrari – cApStAn Ambassador to the Global Village
Slator has been monitoring research related to neural machine translation (NMT) for a number of years. They have recently said that research output has consistently increased since 2014 and more than doubled in 2018 compared to 2017. They base their findings on the number of papers submitted to the Cornell University portal arXiv.org. Interestingly, several large tech companies are focusing on the challenge posed by so-called low-resource languages, i.e. those for which there is little training data (small amounts of parallel texts).
NAIST Japan has experimented augmenting incomplete training data with multiple language sources. Examples of this include the multilingual document collections of the European institutions and the UN, where it is mandatory to (manually) translate all official papers into all the official languages of the organizations. Other sources cited in the paper are “multilingual captions” such as those of talks and movies, based on “voluntary translation efforts”. https://arxiv.org/pdf/1810.06826.pdf
Chinese e-commerce giant Alibaba has trained a NMT system with image descriptions in multiple languages. Their assumption is that the description of the same visual content by different languages should be approximately similar. Their research paper notes that image has become an important source for humans to learn and acquire knowledge so that “the visual signal might be able to disambiguate certain semantics”. They found that combining image with narrative descriptions that can be self-explainable gave better results. https://arxiv.org/pdf/1811.11365.pdf
Microsoft is looking into what is called “transfer learning” to see if this can be applied to low-resource situations. In transfer learning there is an “assisting” source language, just like there are “relay languages” in a number of international institutions. For example, it is possible to find parallel corpora between English and some Indian languages, but very little parallel corpora between Indian languages. Hence, the paper says, it is natural to use English as an assisting language for inter-Indian language translation. However, transfer learning poses a number of issues, including word order divergence, which can create inconsistencies. Pre-ordering the assisting language to match the word order of the source language significantly improved the quality of the translation. https://arxiv.org/pdf/1811.00383.pdf
Read more at: https://slator.com/technology/corporates-going-all-in-on-neural-machine-translation-research/
Photo credit: Persnickety Prints @Unsplash