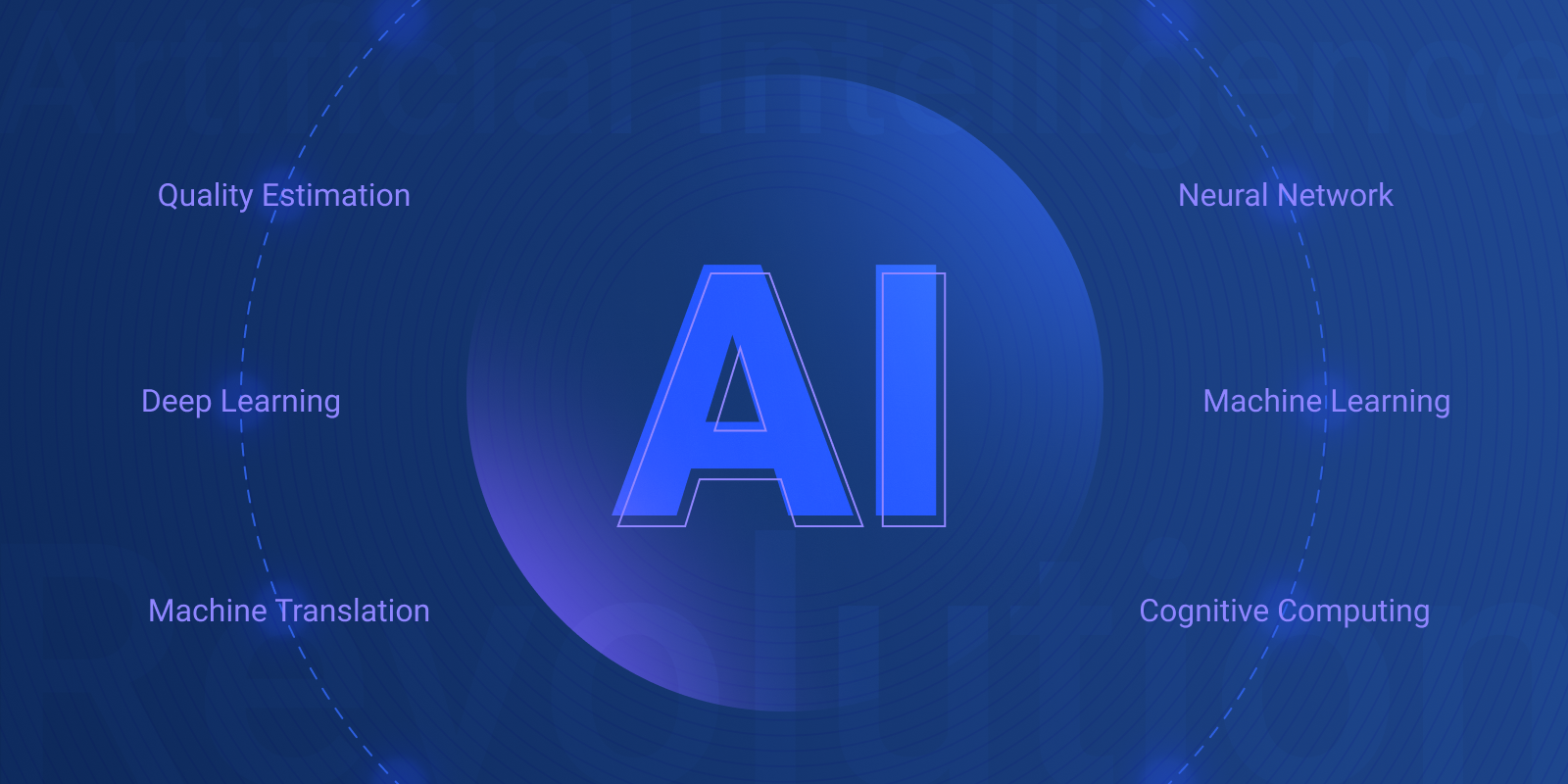
Quality Estimation (AIQE), A New Paradigm
Artificial Intelligence (AI) has been used in translation workflows for quite a while as a way of enhancing productivity by reducing translation effort. In combination with the previous productivity enhancing solution: translation memories (TM), the results are very powerful as they can reduce delivery time and costs significantly. In the 1990s, the introduction of translation memories gave room for a significant decrease in translation costs as, depending on the level of similarity of the TM segment compared with the original source segment, such segments or fuzzy matches, would be paid at a lower rate.
The level of similarity of matches coming from translation memories is predictable and that leads to predictable rates that can be easily agreed by all the parties.
A degree of unpredictability
The introduction of machine translation brought new gains and opportunities, but also a degree of unpredictability.
For many years, translation and localization practitioners have been wishing there was a way or solution that would produce accurate information regarding the level of quality of a machine translated (MT) segment, with the aim of predicting the effort and the cost. During the last decade, the localization industry has relied on analysis performed once the machine translated content had been post-edited, like Edit Distance or Productivity analysis.
- Edit Distance indicates the percentage of content that required post-editing, therefore, the amount of effort required to post-edit a piece of content.
- Productivity analysis indicates the number of words processed per hour (throughputs) by the post-editor.
Such methods, even though very useful and eye opening, help to calculate standard discounted rates for future projects with some degree of accuracy, depending on how close the new content was to the previous one (same language pairs, similar domain, similar level of terminology, etc).
AI Quality Estimation (AIQE)
After this long wait, a technology that calculates the level of quality of the MT output upfront has become available; it is called Quality Estimation or AIQE and is it produced by language models trained specifically for the purpose of estimating levels of quality on the fly without having to rely on reference content.
In a production workflow, quality estimation of machine translation takes place immediately after AI/MT translation and aims to indicate its level of quality. Here are some situations in which quality estimation can become useful:
- To discern if a piece of AI-translated content, for instance a wiki article, has enough quality to be understood without being edited further by a linguist.
- To be able to translate large quantities of research content and limit post-editing to the segments or phrases whose quality is not good enough to be fully understood. For instance, in the case of questionnaires with open questions.
In both cases, the goal of the translation output is simply to be understood, as such pieces of content would be used for gisting purposes (to get an idea of the overall meaning of the content), but there are other cases where the quality expectation are higher; the aim is what it is referred in the industry as publishable quality. To obtain such end quality, all the machine translated content needs to be fully reviewed by an expert post-editor, but still in this case, AI quality estimation has a role to play :
- To divide the AI-translated content into high-confidence and low-confidence segments; whether the high-confidence segments will require a lesser effort and will be paid at a lesser rate.
To take an example, in cApStAn, we have set up this workflow in place for one of our main clients; to translate internal training content into 5 different languages using a combination of translation memories, machine translation, and quality estimation. The volume was substantial and we knew, given the simplicity of the source, that the quality of machine translation was going to be high. In order to establish the point or threshold between the high confidence and low confidence scores, we performed an analysis of the edit distance of an initial 10,000 words and we checked their correlation with the Quality Estimation (AIQE) scores. This method is not bulletproof and requires refinement, but it worked in this case, as we were able to offer our client further savings by dividing the content into two different discounted rates.
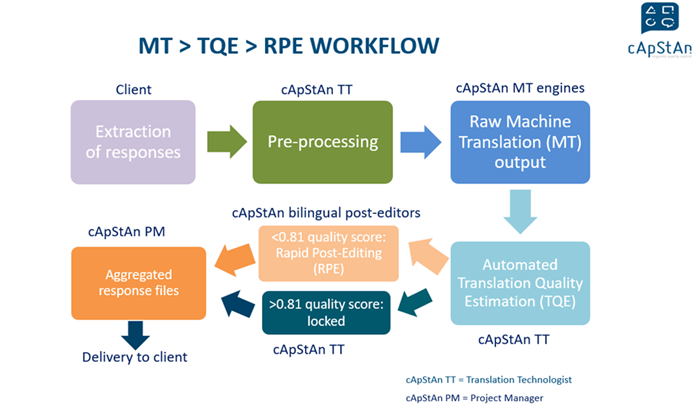
The prediction of quality signifies a change of paradigm within the area of Machine Translation and, even though this technology is only just starting, it is becoming more present in these types of workflows.
In cApStAn we use Quality Estimation in most of our machine translation workflows; if you are interested to hear more about it, get in touch!
Picture Credit: Taus